Midterm 01
Practice problems for topics on Midterm 01.
See Sample Midterm 01 for an example midterm from a previous quarter. This is a good way to get a sense of the format of the exam. However, note that the topics covered may change slightly from quarter to quarter, and so the sample exam may not be a perfect match for the topics covered in this quarter.
Tags in this problem set:
Problem #001
Tags: nearest neighbor
Consider the data set shown below:
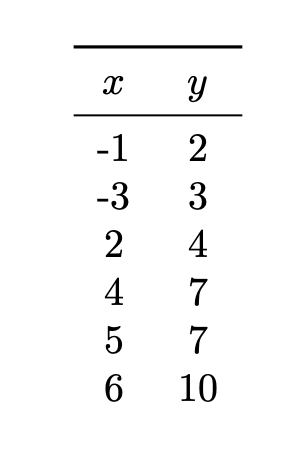
What is the predicted value of \(y\) at \(x = 3\) if the 3-nearest neighbor rule is used?
Solution
6
Problem #002
Tags: linear prediction functions
Suppose a linear prediction rule \(H(\vec x; \vec w) = \Aug(\vec x) \cdot\vec w\) is parameterized by the weight vector \(\vec w = (3, -2, 5, 2)^T\). Let \(\vec z = (1, -1, -2)^T\). What is \(H(\vec z)\)?
Solution
-8
Problem #003
Tags: linear regression, least squares
Suppose a line of the form \(H(x) = w_0 + w_1 x\) is fit to a data set of points \(\{(x_i, y_i)\}\) in \(\mathbb R^2\) by minimizing the mean squared error. Let the mean squared error of this predictor with respect to this data set be \(E_1\).
Next, create a new data set by adding a single new point to the original data set with the property that the new point lies exactly on the line \(H(x) = w_0 + w_1 x\) that was fit above. Let the mean squared error of \(H\) on this new data set be \(E_2\).
Which of the following is true?
Solution
\(E_1 > E_2\)
Problem #004
Tags: linear prediction functions
Suppose a linear predictor \(H_1\) is fit on a data set \(X = \{\nvec{x}{i}, y_i\}\) of \(n\) points by minimizing the mean squared error, where each \(\nvec{x}{i}\in\mathbb R^d\).
Let \(Z = \{\nvec{z}{i}, y_i\}\) be the data set obtained from the original by standardizing each feature. That is, if a matrix were created with the \(i\)th row being \(\nvec{z}{i}\), then the mean of each column would be zero, and the variance would be one.
Suppose a linear predictor \(H_2\) is fit on this standardized data by minimizing the mean squared error.
True or False: \(H_1(\nvec{x}{i}) = H_2(\nvec{z}{i})\) for each \(i = 1, \ldots, n\).
Solution
True.
Problem #005
Tags: object type
Part 1)
Let \(\Phi\) be an \(n \times d\) design matrix, let \(\lambda\) be a real number, and let \(I\) be a \(d \times d\) identity matrix.
What type of object is \((\Phi^T \Phi + n \lambda I)^{-1}\)?
Solution
A \(d \times d\) matrix
Part 2)
Let \(\Phi\) be an \(n \times d\) design matrix, and let \(\vec y \in\mathbb R^n\). What type of object is \(\Phi^T \vec y\)?
Solution
A vector in \(\mathbb R^d\)
Part 3)
Let \(\vec w \in\mathbb R^{d+1}\), and for for each \(i \in\{1, 2, \ldots, n\}\) let \(\nvec{x}{i}\in\mathbb R^d\) and \(y_i \in\mathbb R\).
What type of object is:
Solution
A scalar
Part 4)
Let \(\vec w \in\mathbb R^{d+1}\), and for for each \(i \in\{1, 2, \ldots, n\}\) let \(\nvec{x}{i}\in\mathbb R^d\) and \(y_i \in\mathbb R\). Consider the empirical risk with respect to the square loss of a linear predictor on a data set of \(n\) points:
What type of object is \(\nabla R(\vec w)\); that is, the gradient of the risk with respect to the parameter vector \(\vec w\)?
Solution
A vector in \(\mathbb R^{d+1}\)
Problem #006
Tags: linear prediction functions
Let \(\nvec{x}{1} = (-1, -1)^T\), \(\nvec{x}{2} = (1, 1)^T\), and \(\nvec{x}{3} = (-1, 1)^T\). Suppose \(H\) is a linear prediction function, and that \(H(\nvec{x}{1}) = 2\) while \(H(\nvec{x}{2}) = -2\) and \(H(\nvec{x}{3}) = 0\).
Let \(\nvec{x}{4} = (1,-1)^T\). What is \(H(\nvec{x}{4})\)?
Solution
0
Problem #007
Tags: computing loss
Consider the data set shown below. The ``\(\times\)'' points have label +1, while the ``o'' points have label -1. Shown are the places where a linear prediction function \(H\) is equal to zero, 1, and -1.
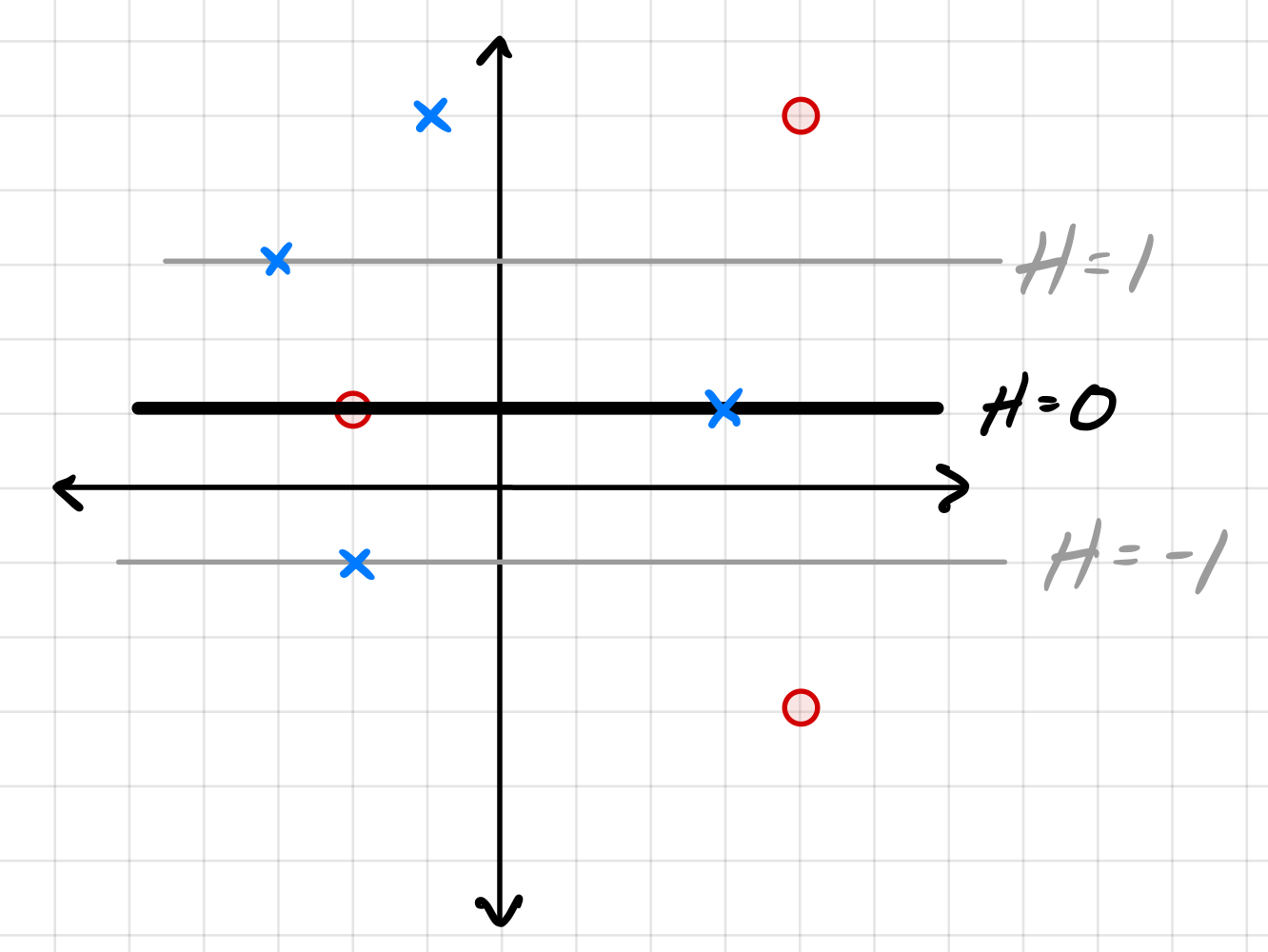
For each of the below subproblems, calculate the total loss with respect to the given loss function. That is, you should calculate \(\sum_{i=1}^n L(\nvec{x}{i}, y_i, \vec w)\) using the appropriate loss function in place of \(L\). Note that we have most often calculated the mean loss, but here we calculate the total so that we encounter fewer fractions.
Part 1)
What is the total square loss of \(H\) on this data set?
Solution
17
Part 2)
What is the total perceptron loss of \(H\) on this data set?
Solution
3
Part 3)
What is the total hinge loss of \(H\) on this data set?
Solution
7
Problem #008
Tags: subgradients
Consider the function \(f(x,y) = x^2 + |y|\). Plots of this function's surface and contours are shown below.
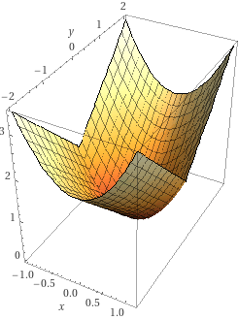
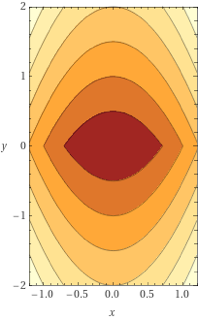
Which of the following are subgradients of \(f\) at the point \((0, 0)\)? Check all that apply.
Solution
\((0, 0)^T\), \((0, 1)^T\), and \((0, -1)^T\) are subgradients of \(f\) at \((0, 0)\).
Problem #009
Tags: gradient descent, perceptrons, gradients
Suppose gradient descent is to be used to train a perceptron classifier \(H(\vec x; \vec w)\) on a data set of \(n\) points, \(\{\nvec{x}{i}, y_i \}\). Recall that each iteration of gradient descent takes a step in the opposite direction of the ``gradient''.
Which gradient is being referred to here?
Solution
The gradient of the empirical risk with respect to \(\vec w\)
Problem #010
Tags: convexity
Let \(\{\nvec{x}{i}\}\) be a set of \(n\) vectors in \(\mathbb R^d\). Consider the function \(f(\vec w) = \sum_{i=1}^n \vec w \cdot\nvec{x}{i}\), where \(\vec w \in\mathbb R^d\).
True or False: \(f\) is convex as a function of \(\vec w\).
Solution
True.
Problem #011
Tags: SVMs
Let \(X = \{\nvec{x}{i}, y_i\}\) be a data set of \(n\) points where each \(\nvec{x}{i}\in\mathbb R^d\).
Let \(Z = \{\nvec{z}{i}, y_i\}\) be the data set obtained from the original by standardizing each feature. That is, if a matrix were created with the \(i\)th row being \(\nvec{z}{i}\), then the mean of each column would be zero, and the variance would be one.
Suppose that \(X\) and \(Z\) are both linearly-separable. Suppose Hard-SVMs \(H_1\) and \(H_2\) are trained on \(X\) and \(Z\), respectively.
True or False: \(H_1(\nvec{x}{i}) = H_2(\nvec{z}{i})\) for each \(i = 1, \ldots, n\).
Solution
False.
This is a tricky problem! For an example demonstrating why this is False, see: https://youtu.be/LSr55vyvfb4
Problem #012
Tags: least squares
Suppose a data set \(\{\nvec{x}{i}, y_i\}\) is linearly-separable.
True or false: a least squares classifier trained on this data set is guaranteed to achieve a training error of zero.
Solution
False
Problem #013
Tags: SVMs
The image below shows a linear prediction function \(H\) along with a data set; the ``\(\times\)'' points have label +1 while the ``\(\circ\)'' points have label -1. Also shown are the places where the output of \(H\) is 0, 1, and -1.
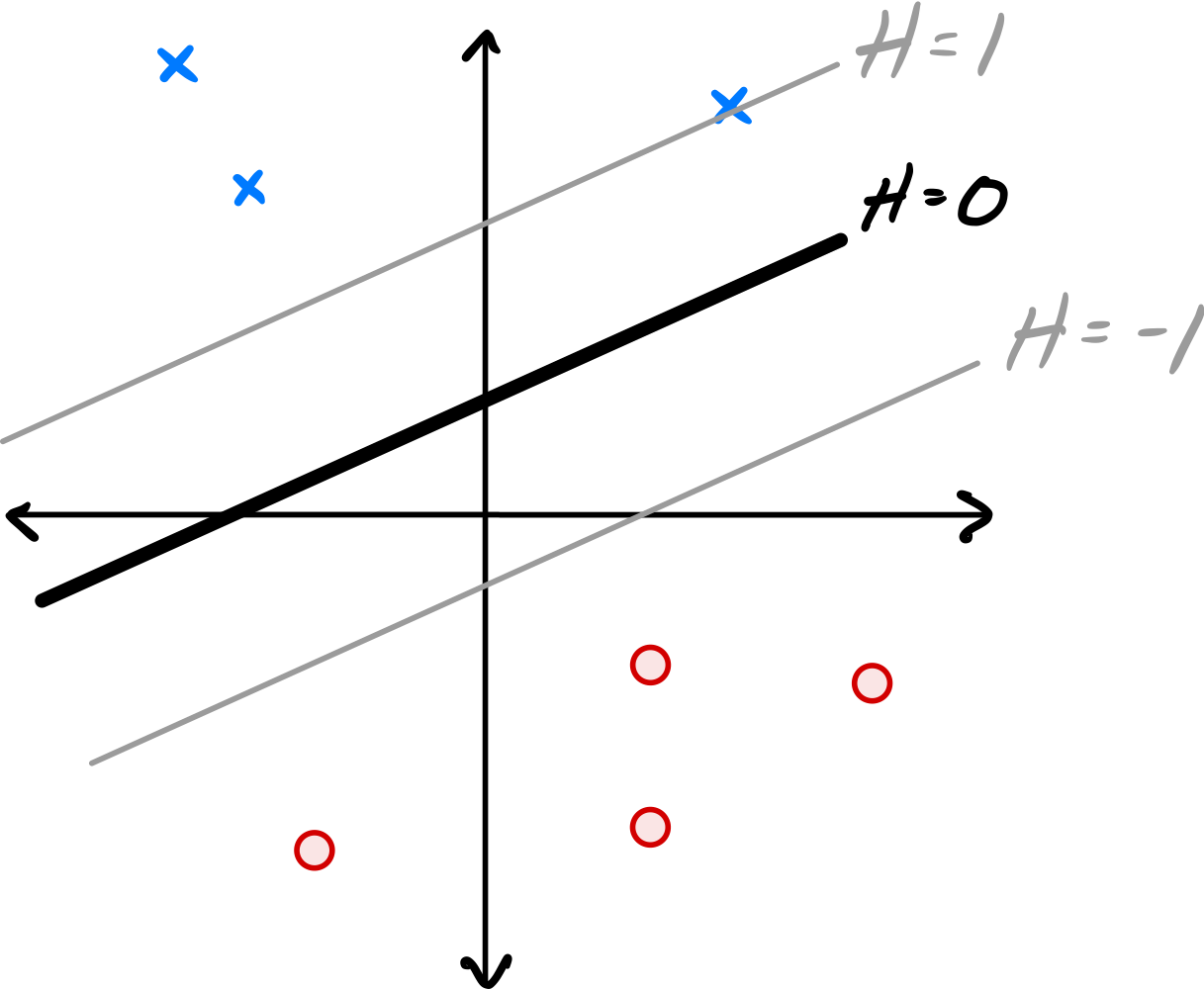
True or False: \(H\) could have been learned by training a Hard-SVM on this data set.
Solution
False.
The solution to the Hard-SVM problem is a hyperplane that separates the two classes with the maximum margin.
In this solution, the margin is not maximized: There is room for the ``exclusion zone'' between the two classes to grow, and for the margin to increase.
Problem #017
Tags: gradients
Let \(f(\vec w) = \vec a \cdot\vec w + \lambda\|\vec w \|^2\), where \(\vec w \in\mathbb R^d\), \(\vec a \in\mathbb R^d\), and \(\lambda > 0\).
What is the minimizer of \(f\)? State your answer in terms of \(\vec a\) and \(\lambda\).
Solution
\(\vec w^* = -\frac{\vec{a}}{2\lambda}\)
Problem #018
Tags: nearest neighbor
Consider the data set of diamonds and circles shown below. Suppose a \(k\)-nn classifier is used to predict the label of the new point marked by \(\times\), with \(k = 3\). What will be the prediction? You may assume that the Euclidean distance is used.
Solution
The new point will be classified as a Diamond.
To classify a point with \(k\)-nn, we examine the \(k\)(in this case, \(k = 3\)) nearest "neighbors" to whichever point we want to classify. Then, whichever class appears more among those neighbors, we'll predict our new point is part of that class too.
So, what are the three neighbors closest to our new point? Since we assume Euclidean distance (and not some other type of distance, like Manhattan distance), this problem becomes fairly easy to solve from first glance.
Graphically: the three nearest points are the red Diamond to the upper-right of our new point, the blue Circle to the lower-left of our new point, and the red Diamond directly above our new point. The diamond class is more common among the three neighbors, so we predict our new point will also be of the Diamond class.
Mathematically: Euclidean distance is defined by \(D = \sqrt{(\delta x)^2 + (\delta y)^2}\), where \(\delta x\) is change in the x-direction and \(\delta y\) is change in the y-direction. We can find the Euclidean distances from each point in our graph to our new point. Whichever three points have the smallest Euclidean distances are the three neighbors we should look at the classes of. After finding all Euclidean distances, we find that the three neighbors we should choose are the same as the ones we chose above, in the visual explanation. The respective Euclidean distances for these three points are \(\sqrt{1^2 + 1^2} = \sqrt{2}\), \(\sqrt{(-1)^2 + (-2)^2} = \sqrt{5}\), and \(\sqrt{0^2 + 3^2} = 3\), which are shorter than all other distances to the new point in the graph. As an aside, the idea behind \(k\)-nn is that we assume a property that if two points are similar in location (i.e. they are near each other), then they are likely similar in class. So, \(k\)-nn is valuable in situations where we have data that is very spatially correlated (ex: predicting price of a new house as "high" or "low" depending on other house prices in the area.)
Solution
To classify a point with \(k\)-nn, we examine the \(k\)(in this case, \(k = 3)\) nearest neighbors to whichever point we want to classify. Then, whichever class appears more among those neighbors, we'll predict our new point is part of that class too.
Graphically: The three nearest points are the red Diamond to the upper-right of our new point, the blue Circle to the lower-left of our new point, and the red Diamond directly above our new point. The diamond class is more common among the three neighbors, so we predict our new point will also be of the Diamond class.
Mathematically: Euclidean distance is defined by \(D = \sqrt{(\delta x)^2 + (\delta y)^2}\), where \(\delta x\) is change in the x-direction and \(\delta y\) is change in the y-direction. We can find the Euclidean distances from each point in our graph to our new point. Whichever three points have the smallest Euclidean distances to our new point are the three neighbors we should look at the classes of. After finding all Euclidean distances, we find that the three neighbors we should choose are the same as the ones we chose above, in the visual explanation. The respective Euclidean distances for these three points to our new point are \(\sqrt{1^2 + 1^2} = \sqrt{2}\), \(\sqrt{(-2)^2 + (-1)^2} = \sqrt{5}\), and \(\sqrt{0^2 + 3^2} = 3\), which are shorter than all other distances to the new point in the graph.
Problem #019
Tags: linear prediction functions
Suppose a linear prediction rule \(H(\vec x; \vec w) = \Aug(\vec x) \cdot\vec w\) is parameterized by the weight vector \(\vec w = (2, 1, -3, 4)^T\). Let \(\vec z = (3, -2, 1)^T\). What is \(H(\vec z)\)?
Solution
15
Problem #020
Tags: least squares
In the following, let \(\mathcal D_1\) be a set of points \((x_1, y_1), \ldots, (x_n, y_n)\) in \(\mathbb R^2\). Suppose a straight line \(H_1(x) = a_1 x + a_0\) is fit to this data set by minimizing the mean squared error, and let \(R_1\) the be mean squared error of this line.
Now create a second data set, \(\mathcal D_2\), by doubling the \(x\)-coordinate of each of the original points, but leaving the \(y\)-coordinate unchanged. That is, \(\mathcal D_2\) consists of points \((2x_1, y_1), \ldots, (2x_n, y_n)\). Suppose a straight line \(H_2(x) = b_1 x + b_0\) is fit to this data set by minimizing the mean squared error, and let \(R_2\) be the mean squared error of this line.
You may assume that all of the \(x_i\) are unique, as are all of the \(y_i\).
Part 1)
Which one of the following is true about \(R_1\) and \(R_2\)?
Solution
\(R_1 = R_2\)
Part 2)
Which one of the following is true about \(a_0\) and \(b_0\)(the intercepts)?
Solution
\(a_0 = b_0\)
Part 3)
Which one of the following is true about \(a_1\) and \(b_1\)(the slopes), assuming that the initial slope is positive?
Solution
\(a_1 > b_1\)
Problem #022
Tags: object type
Part 1)
Let \(\vec x \in\mathbb R^d\) and let \(A\) be an \(d \times d\) matrix. What type of object is \(\vec x^T A \vec x\)?
Solution
A scalar
Part 2)
Let \(A\) be an \(n \times n\) matrix, and let \(\vec x \in\mathbb R^n\). What type of object is: \((A + A^T)^{-1}x\)?
Solution
A vector in \(\mathbb R^n\)
Part 3)
Suppose we train a support vector machine \(H(\vec x) = \Aug(\vec x) \cdot\vec w\) on a data set of \(n\) points in \(\mathbb R^d\). What type of object is the resulting parameter vector, \(\vec w\)?
Solution
A vector in \(\mathbb R^{d+1}\)
Problem #023
Tags: linear prediction functions
Let \(\nvec{x}{1} = (-1, -1)^T\), \(\nvec{x}{2} = (1, 1)^T\), and \(\nvec{x}{3} = (-1,1)^T\). Suppose \(H\) is a linear prediction function, and that \(H(\nvec{x}{1}) = 2\), \(H(\nvec{x}{2}) = -2\), and \(H(\nvec{x}{3}) = 2\).
Let \(\vec z = (2,-1)^T\). What is \(H(\vec z)\)?
Solution
-4
Problem #024
Tags: computing loss
Consider the data set shown below. The ``\(\times\)'' points have label -1, while the ``\(\circ\)'' points have label +1. Shown are the places where a linear prediction function \(H\) is equal to zero (the thick solid line), 1, and -1 (the dotted lines). Each cell of the grid is 1 unit by 1 unit.
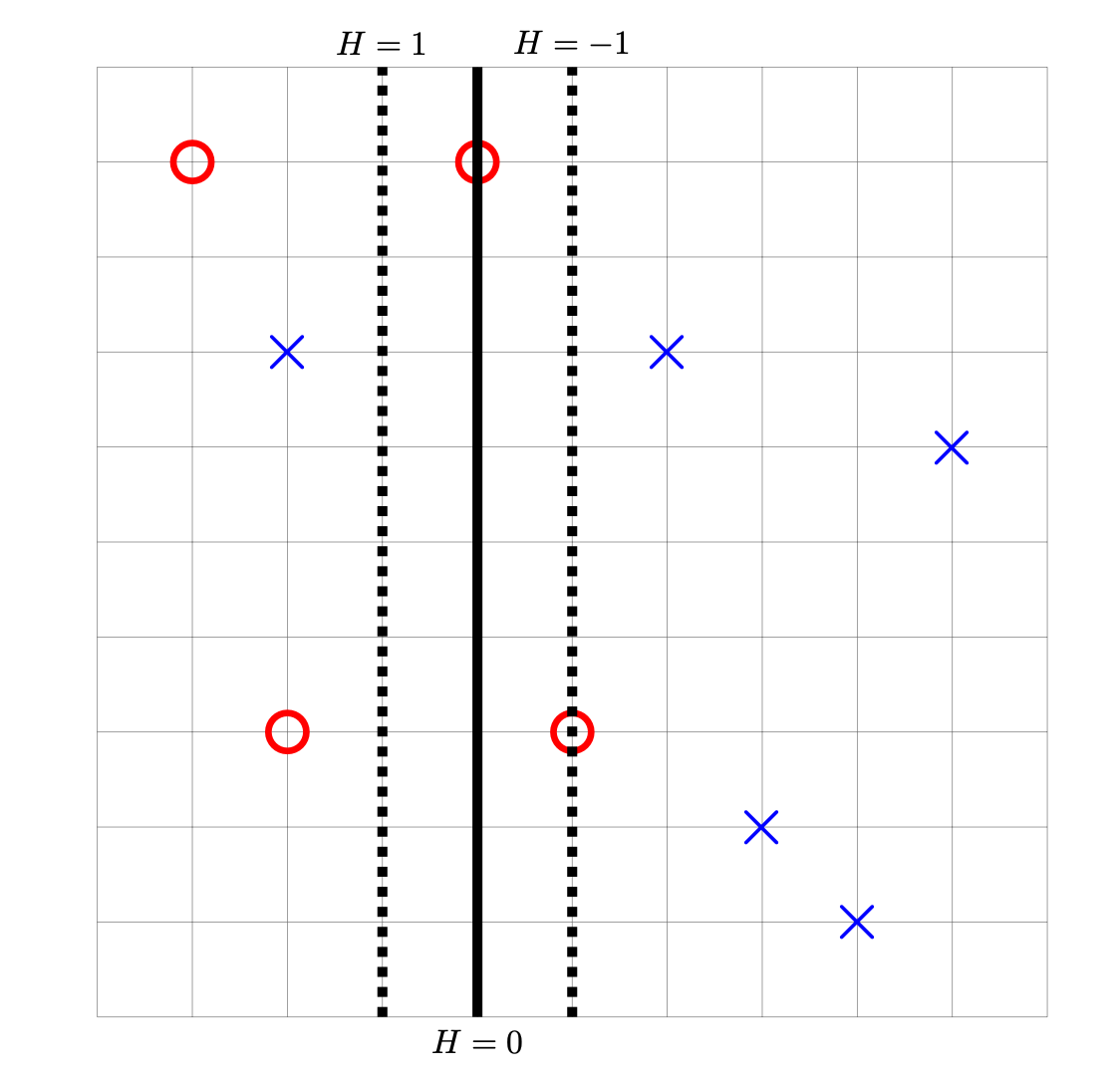
For each of the below subproblems, calculate the total loss with respect to the given loss function. That is, you should calculate \(\sum_{i=1}^n L(\nvec{x}{i}, y_i, \vec w)\) using the appropriate loss function in place of \(L\). Note that we have most often calculated the mean loss, but here we calculate the total so that we encounter fewer fractions.
Part 1)
What is the total square loss of \(H\) on this data set?
Solution
49
Part 2)
What is the total perceptron loss of \(H\) on this data set?
Solution
3
Part 3)
What is the total hinge loss of \(H\) on this data set?
Solution
6
Problem #025
Tags: subgradients
Consider the function \(f(x,y) = |x| + y^4\). Plots of this function's surface and contours are shown below.
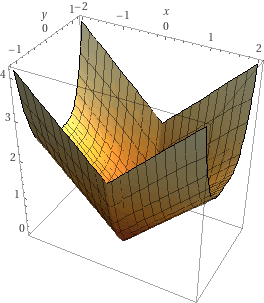
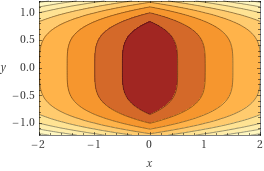
Write a valid subgradient of \(f\) at the point \((0, 1)\).
There are many possibilities, but you need only write one. For the simplicity of grading, please pick a subgradient whose coordinates are both integers, and write your answer in the form \((a, b)\), where \(a\) and \(b\) are numbers.
Solution
\((x, 4)\), where \(x \in[-1,1]\)
Problem #026
Tags: convexity
Consider the function \(f(x) = x^4 - x^2\) True or False: \(f\) is convex as a function of \(x\).
Solution
False.
Problem #027
Tags: SVMs
Suppose a data set \(\mathcal D\) is linearly separable. True or False: a Hard-SVM trained of \(\mathcal D\) is guaranteed to achieve 100\% training accuracy.
Solution
True.
Problem #028
Tags: gradient descent
Consider the function \(f(x, y) = x^2 + xy + y^2\). Suppose that a single iteration of gradient descent is run on this function with a starting location of \((1, 2)^T\) and a learning rate of \(\eta = 1/10\). What will be the \(x\) and \(y\) coordinates after this iteration?
Solution
\(x = 0.6\), \(y = 1.5\)
Problem #029
Tags: SVMs
The image below shows a linear prediction function \(H\) along with a data set; the ``\(\times\)'' points have label +1 while the ``\(\circ\)'' points have label -1. Also shown are the places where the output of \(H\) is 0, 1, and -1.
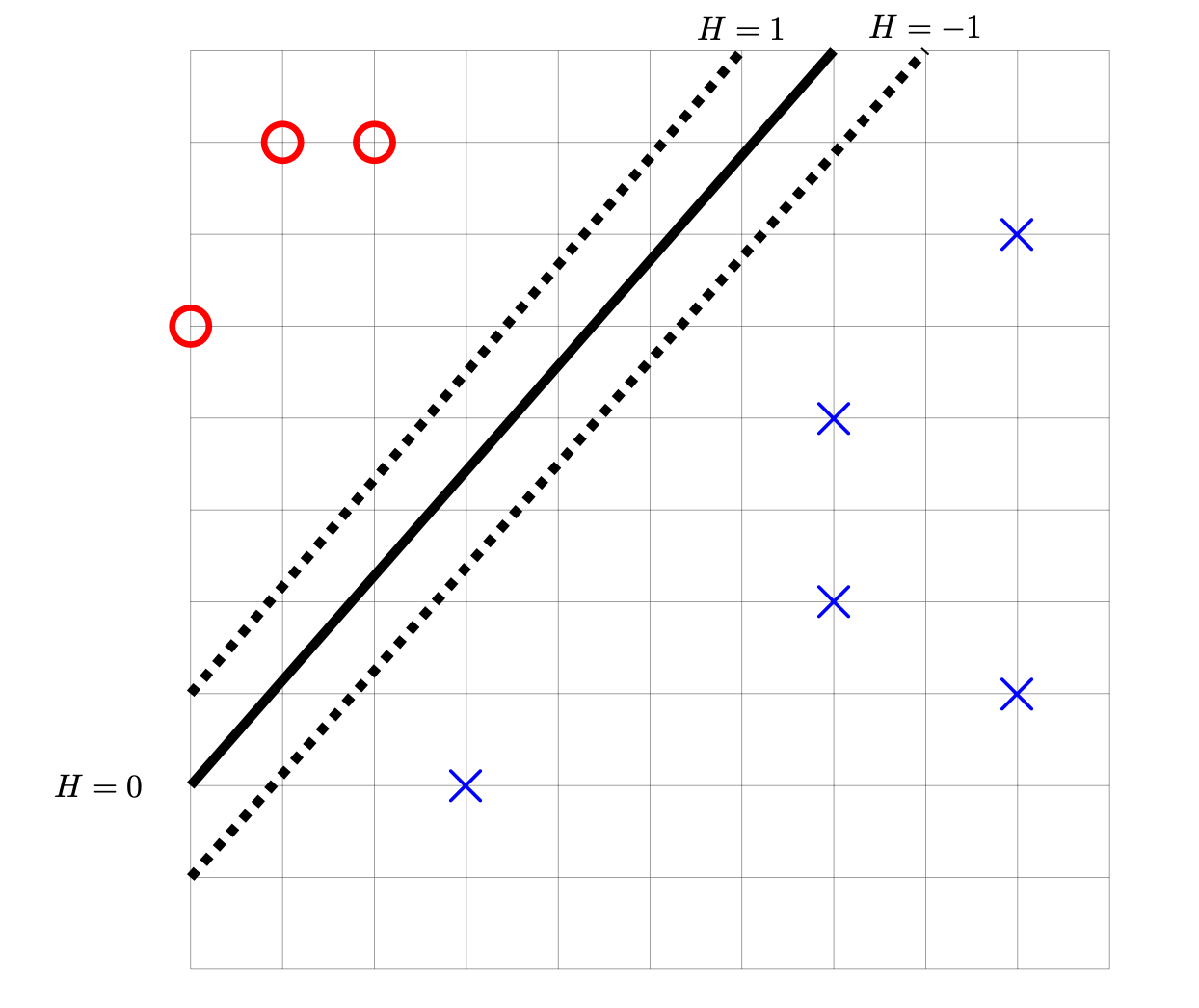
True or False: \(H\) could have been learned by training a Hard-SVM on this data set.
Solution
False.
Problem #059
Tags: nearest neighbor
Let \(\mathcal X = \{(\nvec{x}{1}, y_1), \ldots, (\nvec{x}{n}, y_n)\}\) be a labeled dataset, where \(\nvec{x}{i}\in\mathbb R^d\) is a feature vector and \(y_i \in\{-1, 1\}\) is a binary label. Let \(\vec x\) be a new point that is not in the data set. Suppose a nearest neighbor classifier is used to predict the label of \(\vec x\), and the resulting prediction is \(-1\). (You may assume that there is a unique nearest neighbor of \(\vec x\).)
Now let \(\mathcal Z\) be a new dataset obtained from \(\mathcal X\) by scaling each feature by a factor of 2. That is, \(\mathcal Z = \{(\nvec{z}{1}, y_1), \ldots, (\nvec{z}{n}, y_n)\}\), where \(\nvec{z}{i} = 2 \nvec{x}{i}\) for each \(i\). Let \(\vec z = 2 \vec x\). Suppose a nearest neighbor classifier trained on \(\mathcal Z\) is used to predict the label of \(\vec z\).
True or False: the predicted label of \(\vec z\) must also be \(-1\).
Solution
True.
This question is essentially asking: if we scale a data set by a constant factor, does the nearest neighbor of a point change? The answer is no: therefore, the predicted label cannot change.
When we multiply each feature of a point by the same constant factor, it has the effect of scaling the data set. That is, if \(\mathcal X\) is the data shown in the top of the image shown below, then \(\mathcal Z\) is the data shown in the bottom of the image (not drawn to scale):
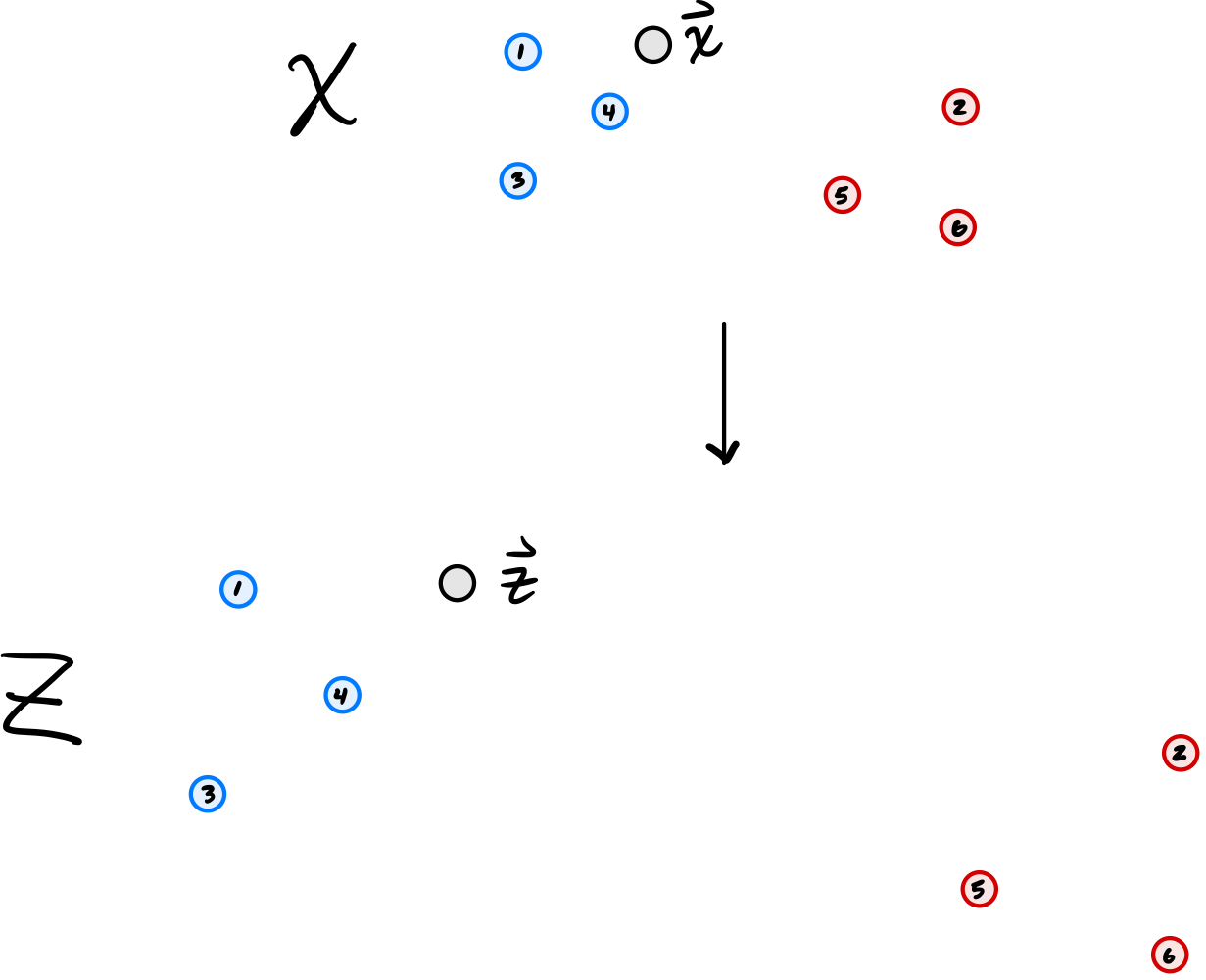
You can see that the nearest neighbor of \(\vec x\) in \(\mathcal X\) is point #4, and the nearest neighbor of \(\vec z\) in \(\mathcal Z\) remains point #4. As such, the predicted label does not change.
Problem #060
Tags: linear prediction functions
Suppose a linear prediction function \(H(\vec x) = w_0 + w_1 x_1 + w_2 x_2\) is trained to perform classification, and the weight vector is found to be \(\vec w = (2, -1, 2)^T\).
The figure below shows four possible decision boundaries: \(A\), \(B\), \(C\), and \(D\). Which of them is the decision boundary of the prediction function \(H\)?
You may assume that each grid square is 1 unit on each side, and the axes are drawn to show you where the origin is.
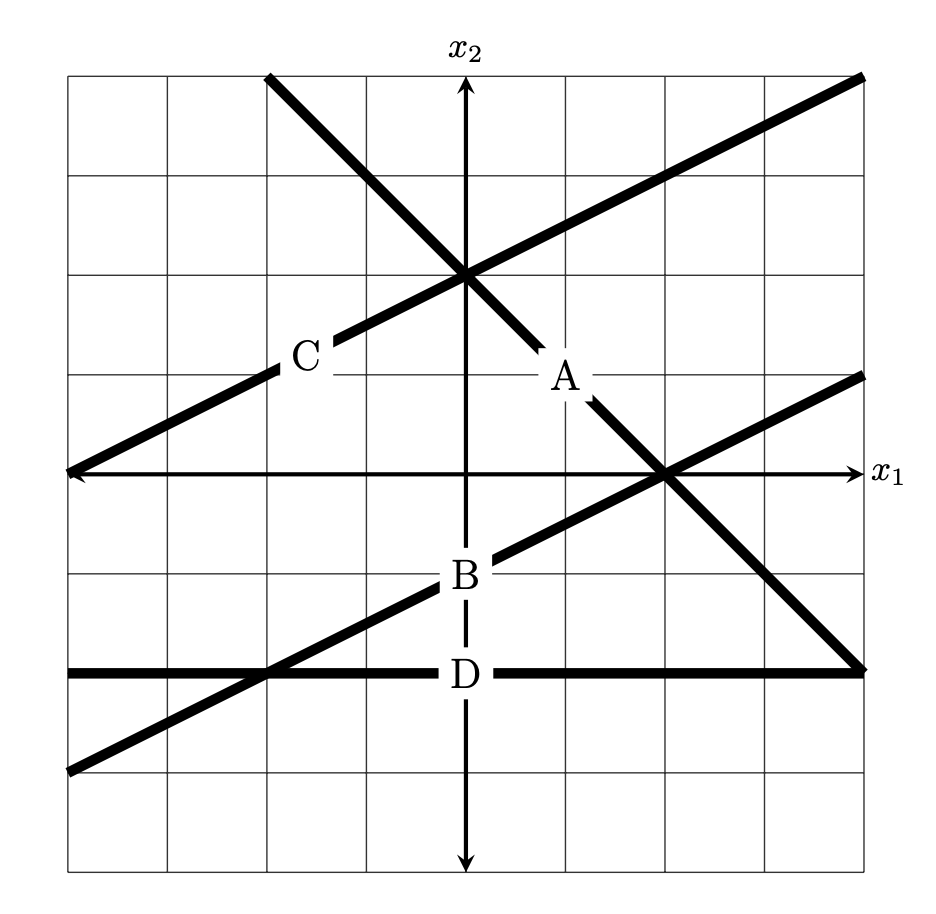
Solution
B.
Here are four ways to solve this problem:
Method 1: Guess and check, using the fact that $H(\vec x)$ must equal zero at every point along the decision boundary. For any of the proposed decision boundaries, we can take a point on the boundary, compute \(H(\vec x)\), and see if it equals 0.
Consider option \(A\), and take an arbitrary point on that boundar -- for example, the point \((1, 1)^T\). Computing \(H(\vec x)\), we get \(H(\vec x) = \vec w \cdot\operatorname{Aug}(\vec x) = (2, -1, 2) \cdot(1, 1, 1) = 3 \neq 0\). Therefore, this cannot be the decision boundary.
Considering option \(B\), it looks like the point \((0, -1)^T\) is on the boundary, Computing the value of \(H\) here, we get \(H(\vec x) = \vec w \cdot\operatorname{Aug}(\vec x) = (2, -1, 2) \cdot(1, -1, 1) = 0\). That's a good sign, but it's not sufficient to say that this must be the decision boundary (at this point, all we can say is that whatever the decision boundary is, it must go through \((0, -1)\).) To make sure, we pick a second point on the boundary, say, \((2, 0)^T\). Computing \(H\) at this point, we get \(H(\vec x) = \vec w \cdot\operatorname{Aug}(\vec x) = (2, -1, 2) \cdot(1, 2, 0) = 0\). With this, we can conclude that \(B\) must be the decision boundary.
Method 2: Use the fact that $(w_1, w_2)$ is orthogonal to the decision boundary. In Homework 02, we learned a useful way of relating the decision boundary to the weight vector \(\vec w = (w_0, w_1, w_2)^T\): it is orthogonal to the vector \(\vec w' = (w_1, w_2)^T\).
In this case, \(\vec w' = (-1, 2)^T\); this is a vector that up and to the left (more up than left). This rules out options \(A\) and \(D\), since they can't be orthogonal to that vector. There are several ways to decide between \(B\) and \(C\), including the logic from Method 1.
Method 3: Find the equation of the decision boundary in $y = mx + b$ form. Another way to solve this problem is to find the equation of the decision boundary in familiar ``\(y = mx + b\)'' form. We can do this by setting \(H(\vec x) = 0\) and solving for \(x_2\) in terms of \(x_1\). We get:
Plugging in \(w_0 = 2\), \(w_1 = -1\), and \(w_2 = 2\), we get
So the decision boundary is a line with slope \(-\frac{1}{2}\) and \(y\)-intercept \(-1\). This is exactly the equation of the line in option \(B\).
Method 4: reasoning about the slope in each coordinate. Similar to Method 2, we can rule out options \(A\) and \(D\) by reasoning about the slope of the decision boundary in each coordinate.
Imagine this scenario: you're standing on the surface of \(H\) at a point where \(H = 0\)(that is, you're on the decision boundary). You will take one step to the right (i.e., one step in the \(x_1\) direction), which will (in general) cause you to leave the decision boundary and either increase or decrease in height. If you want to get back to the decision boundary, and your next step must be either up or down, which should it be? The answer will tell you whether the decision boundary looks like \(/\) or like \(\backslash\).
In this case, taking a step to the right (in the \(x_1\) direction) will cause you to decrease in height. This is because the \(w_1\) component of \(\vec w\) is negative, and so increasing \(x_1\) will decrease \(H\). Now, to get back to the decision boundary, you must increase your height to get back to \(H = 0\). Since the slope in the \(x_2\) direction is positive (2, to be exact), you must increase\(x_2\) in order to increase \(H\). Therefore, the decision boundary is up and to the right: it looks like \(/\).
Problem #061
Tags: feature maps, linear prediction functions
Suppose a linear prediction function \(H(\vec x) = w_1 \phi_1(\vec x) + w_2 \phi_2(\vec x) + w_3 \phi_3(\vec x) + w_4 \phi_4(\vec x)\) is fit using the basis functions
where \(\vec x = (x_1, x_2)^T\) is a feature vector in \(\mathbb R^2\). The weight vector \(\vec w\) is found to be \(\vec w = (1, -2, 3, -4)^T\).
Let \(\vec x = (1, 2)^T\). What is \(H(\vec x)\)?
Solution
We have:
Problem #062
Tags: linear regression, least squares
Suppose a linear regression model \(H(\vec x) = w_0 + w_1 x_1 + w_2 x_2\) is trained by minimizing the mean squared error on the data shown below, where \(x_1\) and \(x_2\) are the features and \(y\) is the target:
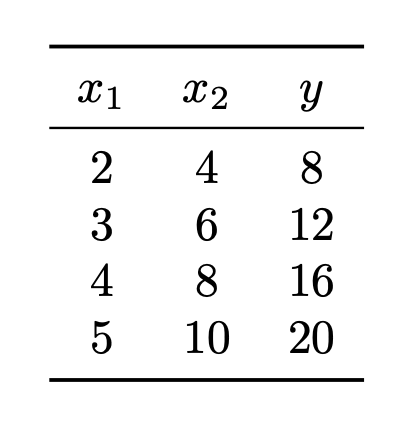
True or False: there is a unique weight vector \(\vec w = (w_0, w_1, w_2)^T\) minimizing the mean squared error for this data set.
Solution
False.
\(y\) can be predicted exactly using only \(x_1\). In particular, \(y = 4x_1\). So the weight vector \(\vec w = (0, 4, 0)^T\) is one that minimizes the mean squared error.
On the other hand, \(y\) can also be predicted exactly using only \(x_2\). In particular, \(y = 2x_2\). So the weight vector \(\vec w = (0, 0, 2)^T\) is another that minimizes the mean squared error.
In fact, there are infinitely many weight vectors that minimize the mean squared error in this case. Geometrically, this is because the data are all along a 2-dimensional line in 3-dimensional space, and by doing regression we are fitting a plane to the data. There are infinitely many planes that pass through a given line, each of them with a different corresponding weight vector, so there are infinitely many weight vectors that minimize the mean squared error.
Now you might be thinking: the mean squared error is convex, so there can be only one minimizer. It is true that the MSE is convex, but that doesn't imply that there is only one minimizer! More specifically, any local minima of a convex function is also a global minimum, but there can be many local minima. Take, for example, the function \(f(x, y) = x^2\). The point \((0, 0)\) is a minimizer, but so is \((0, 2)\) and \((0, 100)\), and so on. There are infinitely many minimizers!
Problem #063
Tags: linear prediction functions
Suppose a linear prediction function \(H(\vec x) = w_0 + w_1 x_1 + w_2 x_2\) is fit to data and the following are observed:
At a point, \(\nvec{x}{1} = (1, 1)^T\), the value of \(H\) is 4.
At a point, \(\nvec{x}{2} = (1, 0)^T\), the value of \(H\) is 0.
At a point, \(\nvec{x}{3} = (0, 0)^T\), the value of \(H\) is -4.
What is the value of \(H\) at the point \(\nvec{x}{4} = (0, 1)^T\)?
Solution
The four points make a square:
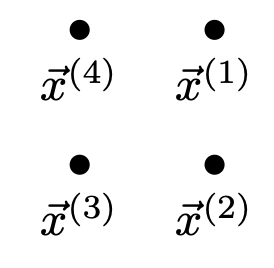
Since \(H = 0\) at \(\nvec{x}{2}\), the decision boundary must pass through that point. And since \(H(\nvec{x}{1}) = 4 = -H(\nvec{x}{3})\), the points \(\nvec{x}{1}\) and \(\nvec{x}{3}\) are equidistant from the decision boundary. Therefore, the decision boundary must pass exactly halfway between \(\nvec{x}{1}\) and \(\nvec{x}{3}\). This makes a line going through \(\nvec{x}{4}\), meaning that \(\nvec{x}{4}\) is on the decision boundary, so \(H(\nvec{x}{4}) = 0\).
Problem #064
Tags: object type
Choose the option which best completes the following sentence: In least squares regression, we can fit a linear prediction function \(H\) by computing the gradient of the _________ with respect to ________ and solving.
Solution
risk, the weights
Problem #065
Tags: object type
Part 1)
For each \(i = 1, \ldots, n\), let \(\nvec{x}{i}\) be a vector in \(\mathbb R^d\) and let \(\alpha_i\) be a scalar. What type of object is:
Solution
A vector in \(\mathbb R^d\)
Part 2)
Let \(\Phi\) be an \(n \times d\) matrix, let \(\vec y\) be a vector in \(\mathbb R^n\), and let \(\vec\alpha\) be a vector in \(\mathbb R^n\). What type of object is:
Solution
A scalar
Part 3)
Let \(\vec x\) be a vector in \(\mathbb R^d\), and let \(A\) be a \(d \times d\) matrix. What type of object is:
Solution
A scalar
Part 4)
Let \(A\) be a \(d \times n\) matrix. What type of object is \((A A^T)^{-1}\)?
Solution
A \(d \times d\) matrix
Part 5)
For each \(i = 1, \ldots, n\), let \(\nvec{x}{i}\) be a vector in \(\mathbb R^d\). What type of object is:
Solution
A \(d \times d\) matrix
Problem #066
Tags: computing loss
Consider the data set shown below. The 4 points marked with ``+'' have label +1, while the 3 ``\(\circ\)'' points have label -1. Shown are the places where a linear prediction function \(H\) is equal to zero (the thick solid line), 1, and -1 (the dotted lines). Each cell of the grid is 1 unit by 1 unit. The origin is not specified; it is not necessary to know the absolute coordinates of the data to complete this problem.
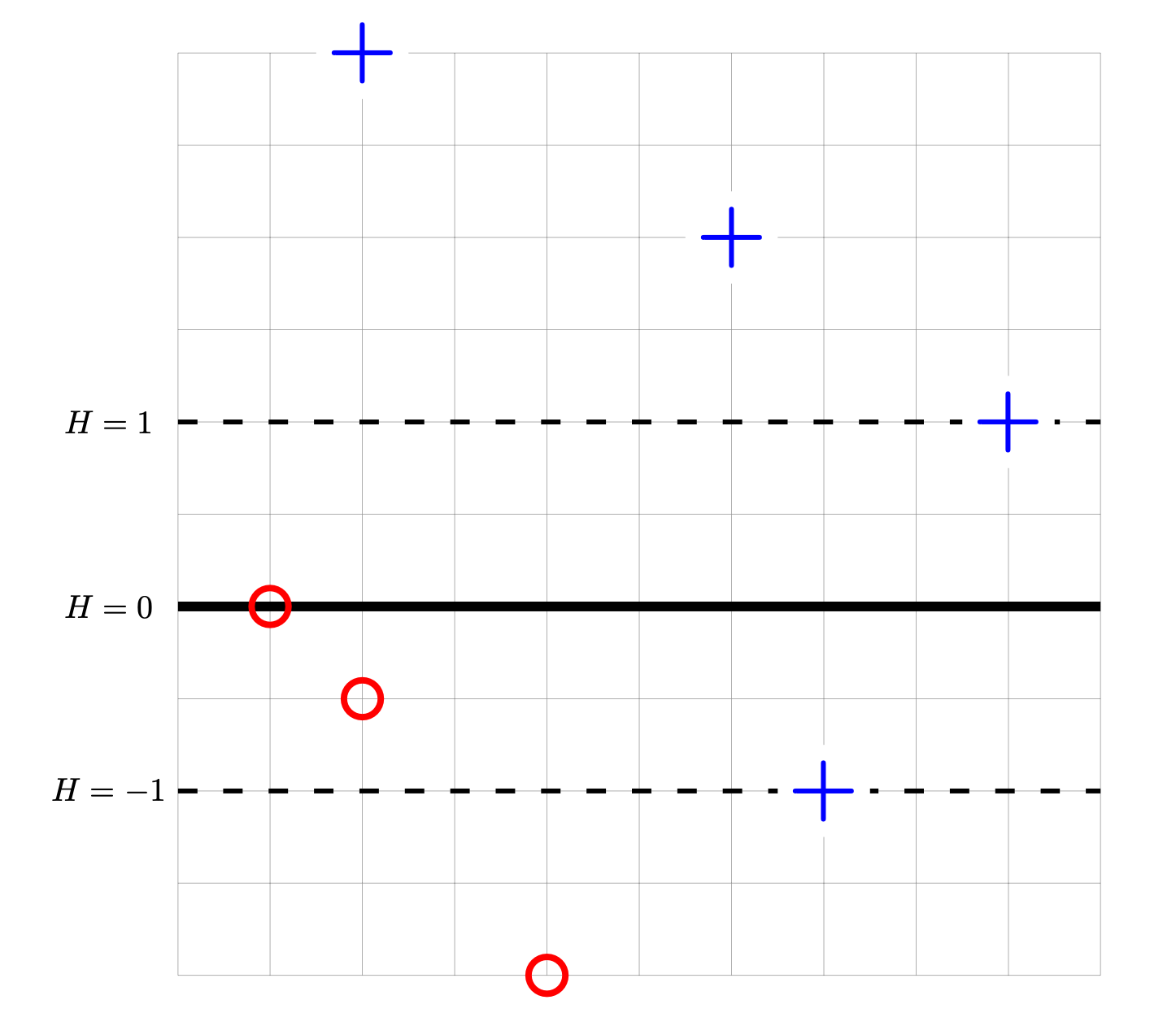
For each of the below subproblems, calculate the total loss with respect to the given loss function. That is, you should calculate \(\sum_{i=1}^n L(\nvec{x}{i}, y_i, \vec w)\) using the appropriate loss function in place of \(L\). Note that we have most often calculated the mean loss, but here we calculate the total so that we encounter fewer fractions.
Part 1)
What is the totalsquare loss of \(H\) on this data set?
Part 2)
What is the totalperceptron loss of \(H\) on this data set?
Part 3)
What is the totalhinge loss of \(H\) on this data set?
Problem #067
Tags: subgradients
Consider the piecewise function:
For convenience, a plot of this function is shown below:
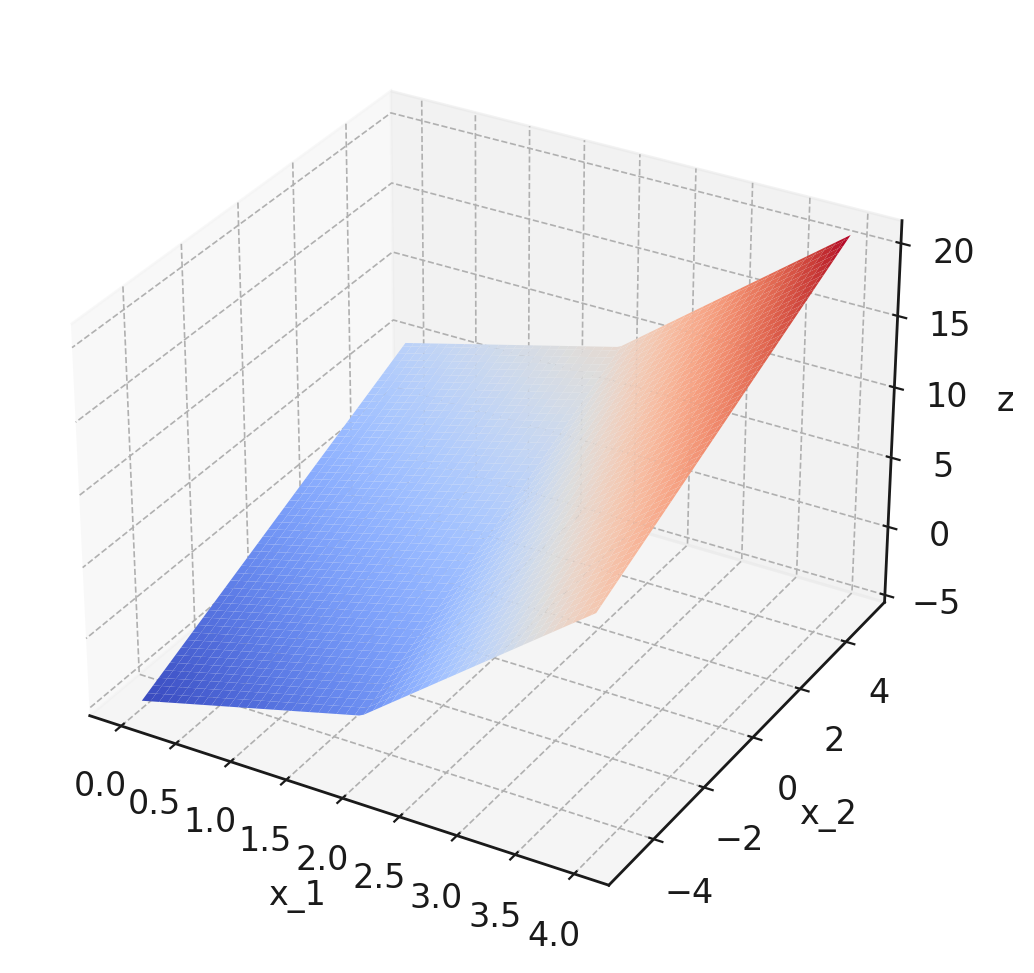
Note that the plot isn't intended to be precise enough to read off exact values, but it might help give you a sense of the function's behavior. In principle, you can do this question using only the piecewise definition of \(f\) given above.
Part 1)
What is the gradient of this function at the point \((0, 0)\)?
Part 2)
Which of the below are valid subgradients of the function at the point \((2,0)\)? Select all that apply.
Solution
\((3,1)^T\) and \((2,1)^T\) are valid subgradients of the function at \((2,0)\).
Problem #068
Tags: gradient descent
Recall that a subgradient of the absolute loss is:
Suppose you are running subgradient descent to minimize the risk with respect to the absolute loss in order to train a function \(H(x) = w_0 + w_1 x\) on the following data set:
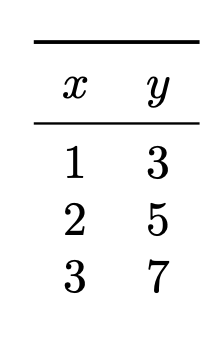
Suppose that the initial weight vector is \(\vec w = (0, 0)^T\) and that the learning rate \(\eta = 1\). What will be the weight vector after one iteration of subgradient descent?
Solution
To perform subgradient descent, we need to compute the subgradient of the risk. The main thing to remember is that the subgradient of the risk is the average of the subgradient of the loss on each data point.
So to start this problem, calculuate the subgradient of the loss for each of the three points. Our formula for the subgradient of the absolute loss tells us to compute \(\Aug(\vec x) \cdot w - y\) for each point and see if this is positive or negative. If it is positive, the subgradient is \(\Aug(\vec x)\); if it is negative, the subgradient is \(-\Aug(\vec x)\).
Now, the initial weight vector \(\vec w\) was conveniently chosen to be \(\vec 0\), meaning that \(\operatorname{Aug}(\vec x) \cdot\vec w = 0\) for all of our data points. Therefore, when we compute \(\operatorname{Aug}(\vec x) \cdot\vec w - y\), we get \(-y\) for every data point, and so we fall into the second case of the subgradient formula for every data point. This means that the subgradient of the loss at each data point is \(-\operatorname{Aug}(\vec x)\). Or, more concretely, the subgradient of the loss at each of the three data points is:
\((-1, -1)^T\)\((-1, -2)^T\)\((-1, -3)^T\) This means that the subgradient of the risk is the average of these three:
The subgradient descent update rule says that \(\vec w^{(1)} = \vec w^{(0)} - \eta\vec g\), where \(\vec g\) is the subgradient of the risk. The learning rate \(\eta\) was given as 1, so we have \(\vec w^{(1)} = \vec w^{(0)} - \vec g = \vec 0 - \begin{bmatrix}-1\\ -2 \end{bmatrix} = \begin{bmatrix}1\\ 2 \end{bmatrix}\).
Problem #069
Tags: convexity
Let \(\mathcal X = \{(\nvec{x}{1}, y_1), \ldots, (\nvec{x}{n}, y_n)\}\) be a data set for regression, with each \(\nvec{x}{i}\in\mathbb R^d\) and each \(y_i \in\mathbb R\). Consider the following function:
True or False: \(R(\vec w)\) is a convex function of \(\vec w\).
Solution
True.
Problem #071
Tags: SVMs
In lecture, we saw that SVMs minimize the (regularized) risk with respect to the hinge loss. Now recall, the 0-1 loss, which was defined in lecture as:
Part 1)
Suppose a Hard SVM is trained on linearly-separable data. True or False: the solution is guaranteed to have the smallest 0-1 risk of any possible linear prediction function \(H(\vec x) = \vec w \cdot\operatorname{Aug}(\vec x)\).
Solution
True. If the data is linearly seperable, then a Hard-SVM will draw a decision boundary that goes between the two classes, perfectly classifying the training data and achieve a 0-1 risk of zero.
Part 2)
Suppose a Soft SVM is trained on linearly-separable data using an unknown slack parameter \(C\). True or False: the solution is guaranteed to have the smallest 0-1 risk of any possible linear prediction function \(H(\vec x) = \vec w \cdot\operatorname{Aug}(\vec x)\).
Solution
False.
Part 3)
Suppose a Soft SVM is trained on data that is not linearly separable using an unknown slack parameter \(C\). True or False: the solution is guaranteed to have the smallest 0-1 risk of any possible linear prediction function \(H(\vec x) = \vec w \cdot\operatorname{Aug}(\vec x)\).
Solution
False.
Problem #072
Tags: perceptrons, gradients
Suppose a perceptron classifier \(H(\vec x) = \vec w \cdot\vec x\) is trained on the data shown below. The points marked with ``+'' have label +1, while the ``\(\circ\)'' points have label -1. The perceptron's decision boundary is plotted as a thick solid line.
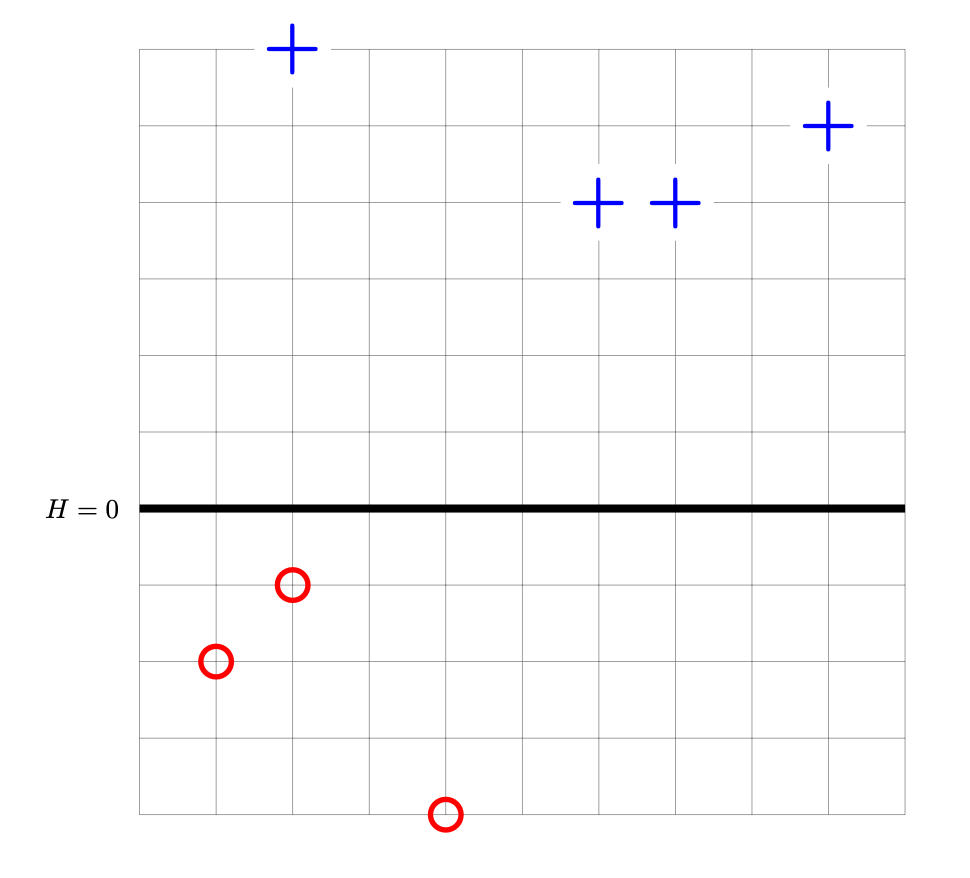
Let \(R(\vec w)\) be the risk with respect to the perceptron loss function, and let \(\vec w^*\) be the weight vector whose corresponding decision boundary is shown above.
True or False: the gradient of \(R\) evaluated at \(\vec w^*\) is \(\vec 0\).
Solution
True.
Problem #073
Tags: SVMs
Consider the data set shown below. The points marked with ``+'' have label +1, while the ``\(\circ\)'' points have label -1. Shown are the places where a linear prediction function \(H\) is equal to zero (the thick solid line), 1, and -1 (the dotted lines). Each cell of the grid is 1 unit by 1 unit. The origin is not specified (it is not necssary to know the absolute coordinates of any points for this problem).
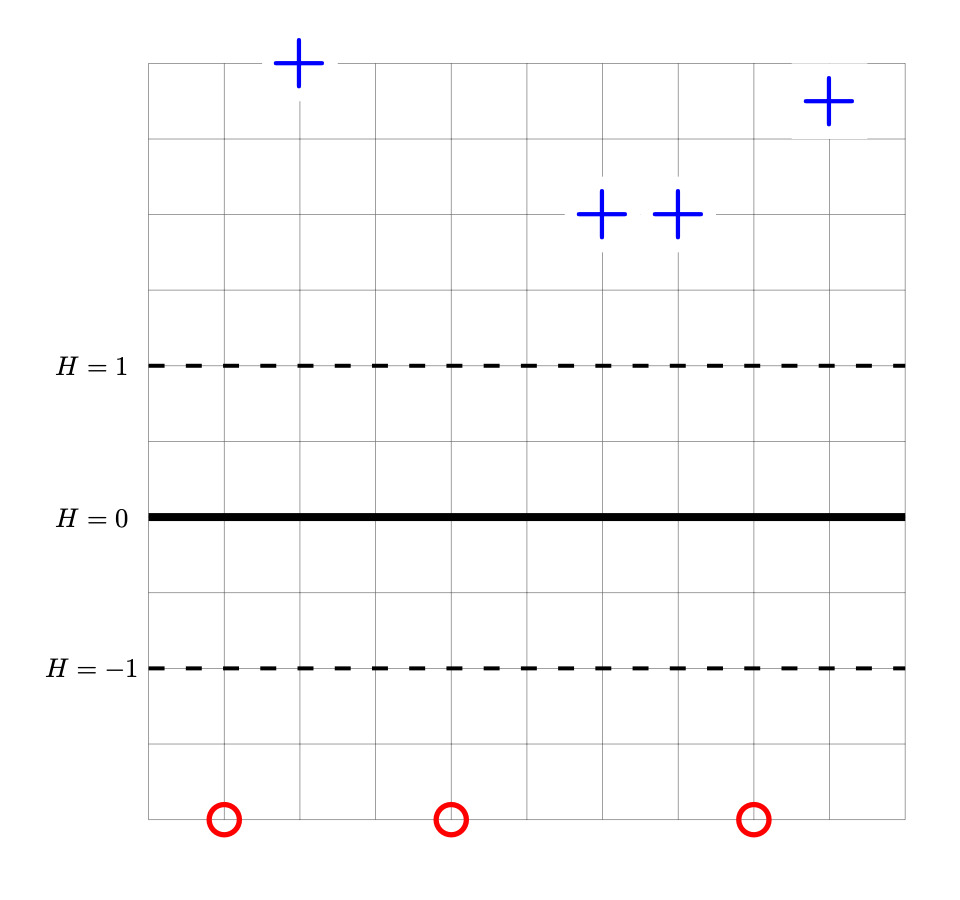
Suppose that the weight vector \(\vec w\) of the linear prediction function \(w_0 + w_1 x_1 + w_2 x_2\) shown above is \((6, 0, \frac{1}{2})^T\). This weight vector is not the solution to the Hard SVM problem.
What weight vector is the solution of the Hard SVM problem for this data set?
Solution
Although this decision boundary is in the right place, it can't be the solution to the Hard-SVM problem because its margin isn't maximized. Remember that the surface of \(H\) is a plane, and in this case the plane is too steep; we need to decrease its slope. We do this by scaling the weight vector by a constant factor; in this case, we want to double the margin, so we need to halve the slope. Therefore, the right answer is \(\frac{1}{2}(6, 0, \frac{1}{2})^T = (3, 0, \frac{1}{4})^T\).
Problem #074
Tags: gradients
Let \(\nvec{x}{1}, \ldots, \nvec{x}{n}\) be vectors in \(\mathbb R^d\) and let \(\vec w\) be a vector in \(\mathbb R^d\). Consider the function:
What is \(\frac{d}{d \vec w} f(\vec w)\)?
Solution
Problem #076
Tags: object type
Part 1)
Let \(f : \mathbb R^d \to\mathbb R\) be a function and let \(\nvec{x}{0}\) be a vector in \(\mathbb R^d\). What type of object is \(\frac{d}{d \vec x} f(\nvec{x}{0})\)? In other words, what type of object is the gradient of \(f\) evaluated at \(\nvec{x}{0}\)?
Solution
A vector in \(\mathbb R^d\).
Part 2)
Let \(\Phi\) be an \(n \times d\) matrix and let \(\vec\alpha\) be a vector in \(\mathbb R^n\). What type of object is:
Solution
A scalar.
Part 3)
For each \(i = 1, \ldots, n\), let \(\nvec{x}{i}\) be a vector in \(\mathbb R^d\) and \(y_i\) be a scalar. Let \(\vec w\) be a vector in \(\mathbb R^d\). What type of object is:
Solution
A scalar.
Part 4)
Let \(X\) be an \(n \times d\) matrix, and assume that \(X^T X\) is invertible. What type of object is \(X(X^T X)^{-1} X^T\)?
Solution
An \(n \times n\) matrix.
Problem #077
Tags: nearest neighbor
Let \(\mathcal X = \{(\nvec{x}{1}, y_1), \ldots, (\nvec{x}{n}, y_n)\}\) be a labeled dataset, where \(\nvec{x}{i}\in\mathbb R^d\) is a feature vector and \(y_i \in\{-1, 1\}\) is a binary label. Let \(\vec x\) be a new point that is not in the data set. Suppose a nearest neighbor classifier is used to predict the label of \(\vec x\), and the resulting prediction is \(-1\). (You may assume that there is a unique nearest neighbor of \(\vec x\).)
Now let \(\mathcal Z\) be a new dataset obtained from \(\mathcal X\) by subtracting the same vector \(\vec\delta\) from each training point. That is, \(\mathcal Z = \{(\nvec{z}{1}, y_1), \ldots, (\nvec{z}{n}, y_n)\}\), where \(\nvec{z}{i} = \nvec{x}{i} - \vec\delta\) for each \(i\). Let \(\vec z = \vec x - \vec\delta\). Suppose a nearest neighbor classifier trained on \(\mathcal Z\) is used to predict the label of \(\vec z\).
True or False: the predicted label of \(\vec z\) must also be \(-1\).
Solution
True.
Problem #078
Tags: linear prediction functions
Suppose a linear prediction function \(H(\vec x) = w_0 + w_1 x_1 + w_2 x_2\) is trained to perform classification, and the weight vector is found to be \(\vec w = (1,-2,1)^T\).
The figure below shows four possible decision boundaries: \(A\), \(B\), \(C\), and \(D\). Which of them is the decision boundary of the prediction function \(H\)?
You may assume that each grid square is 1 unit on each side, and the axes are drawn to show you where the origin is.
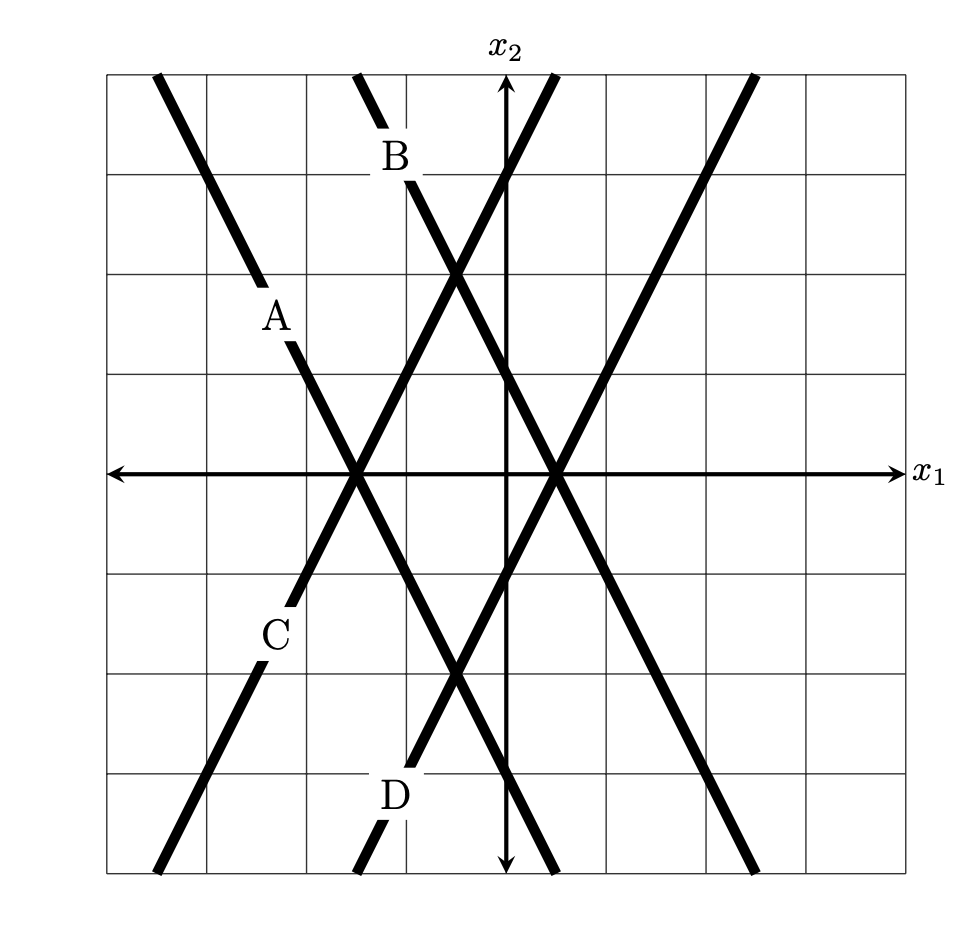
Solution
D.
Problem #079
Tags: feature maps, linear prediction functions
Suppose a linear prediction function \(H(\vec x) = w_1 \phi_1(\vec x) + w_2 \phi_2(\vec x) + w_3 \phi_3(\vec x) + w_4 \phi_4(\vec x)\) is fit using the basis functions
where \(\vec x = (x_1, x_2, x_3)^T\) is a feature vector in \(\mathbb R^3\). The weight vector \(\vec w\) is found to be \(\vec w = (2, 1, -2, -3)^T\).
Let \(\vec x = (1, 2, 1)^T\). What is \(H(\vec x)\)?
Problem #080
Tags: least squares, linear prediction functions
Consider the binary classification data set shown below consisting of six points in \(\mathbb R^2\). Each point has an associated label of either +1 or -1.
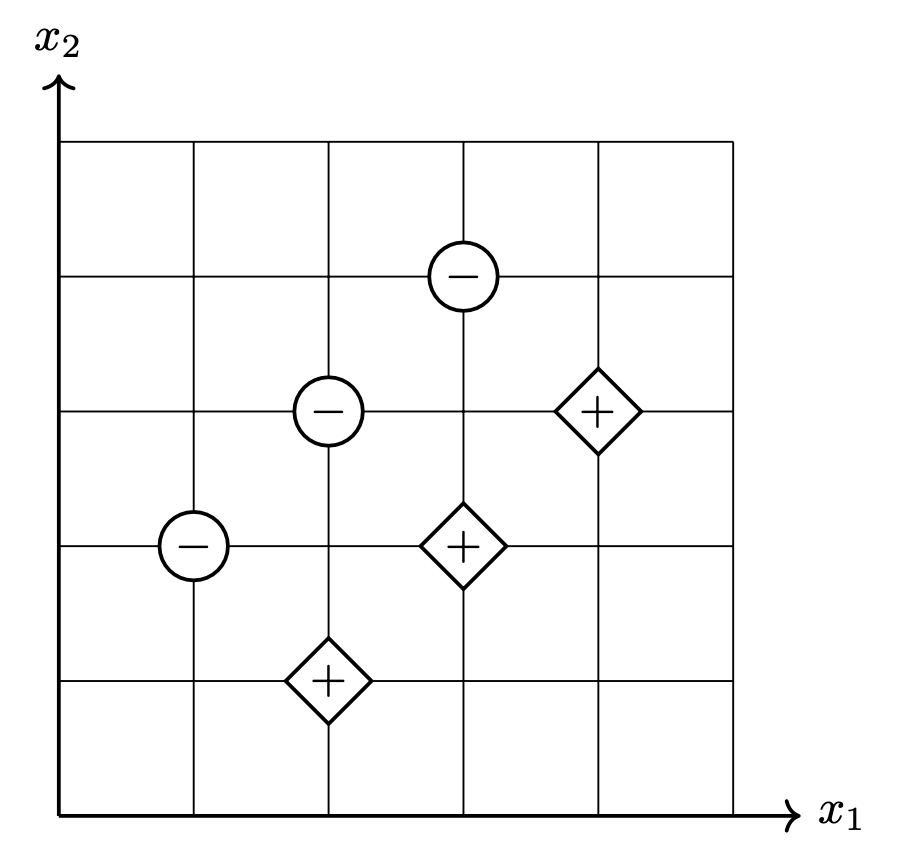
What is the mean squared error of a least squares classifier trained on this data set (without regularization)? Your answer should be a number.
Problem #081
Tags: convexity
Suppose \(f(x_1, x_2)\) is a convex function. Define the new function \(g(x) = f(x, 0)\). True or False: \(g(x)\) must be convex.
Solution
True.
Problem #082
Tags: convexity
Let \(f_1(x)\) be a convex function and let \(f_2(x)\) be a non-convex function. Define the new function \(f(x) = f_1(x) + f_2(x)\). True or False: \(f(x)\) must be non-convex.
Solution
For a simple counterexample, let \(f_1(x) = 4x^2\) and \(f_2(x) = -x^2\). Then \(f(x) = 4x^2 - x^2 = 3x^2\) is convex.
If that example seems too contrived, consider \(f_1(x) = x^4 + 3 x^2\) and \(f_2(x) = x^3\). Then \(f(x) = x^4 + x^3 + 3x^2\), and the second derivative test gives us \(f''(x) = 12x^2 + 6x + 6\), which is positive for all \(x\).
Problem #083
Tags: computing loss
Consider the data set shown below. The \(\diamond\) points marked with ``+'' have label +1, while the \(\circ\) points marked with ``\(-\)'' have label -1. Shown are the places where a linear prediction function \(H\) is equal to zero (the thick solid line), 1, and -1 (the dotted lines). Each cell of the grid is 1 unit by 1 unit. The origin is not specified; it is not necessary to know the absolute coordinates of the data to complete this problem.
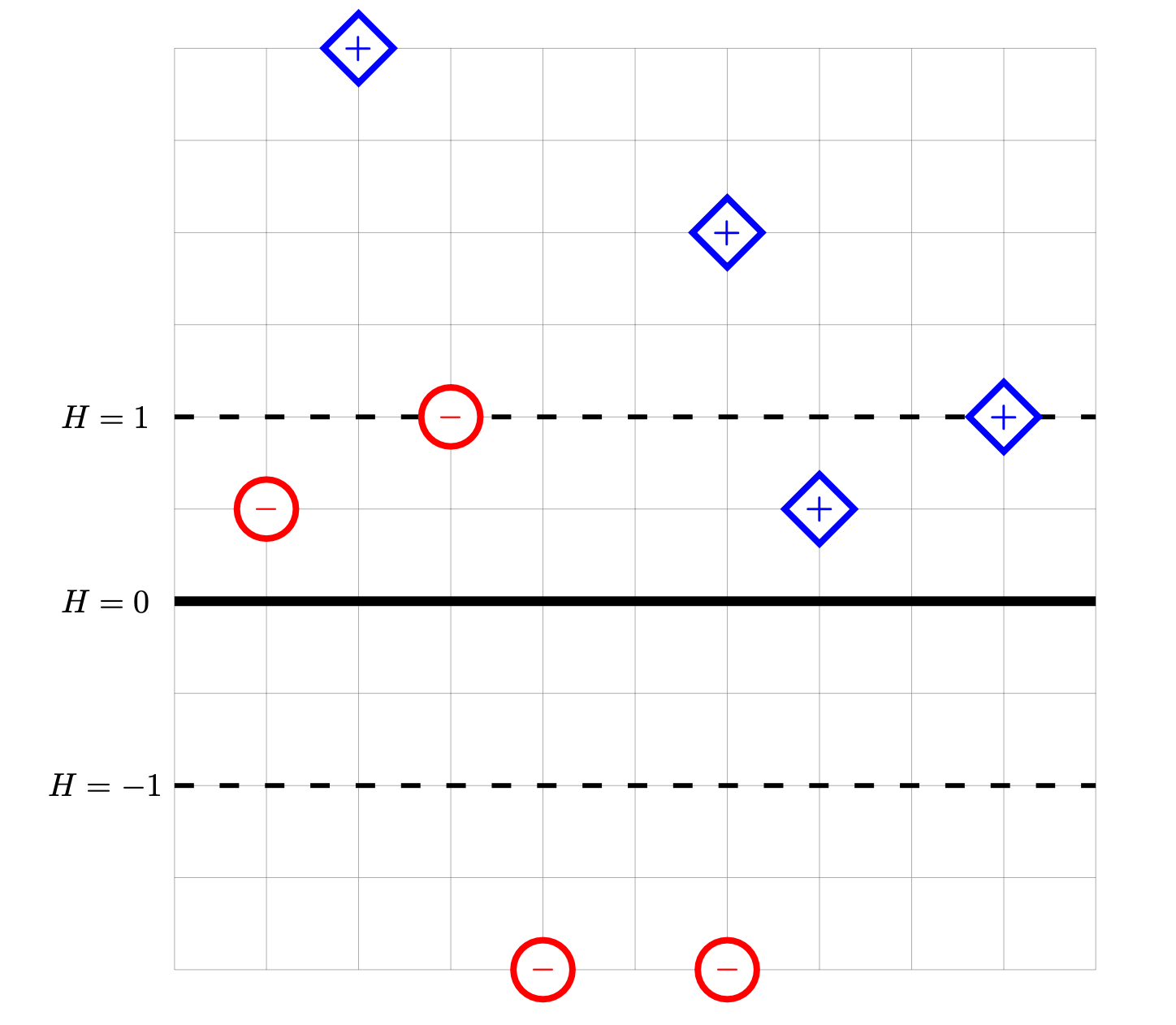
For each of the below subproblems, calculate the total loss with respect to the given loss function. That is, you should calculate \(\sum_{i=1}^n L(\nvec{x}{i}, y_i, \vec w)\) using the appropriate loss function in place of \(L\). Note that we have most often calculated the mean loss, but here we calculate the total so that we encounter fewer fractions.
Note: please double-check your calculations! We are not grading your work, so partial credit is difficult to assign on this problem.
Part 1)
What is the totalsquare loss of \(H\) on this data set?
Part 2)
What is the totalperceptron loss of \(H\) on this data set?
Part 3)
What is the totalhinge loss of \(H\) on this data set?
Problem #084
Tags: subgradients, gradients
Consider the following piecewise function that is linear in each quadrant:
For convenience, a plot of this function is shown below:
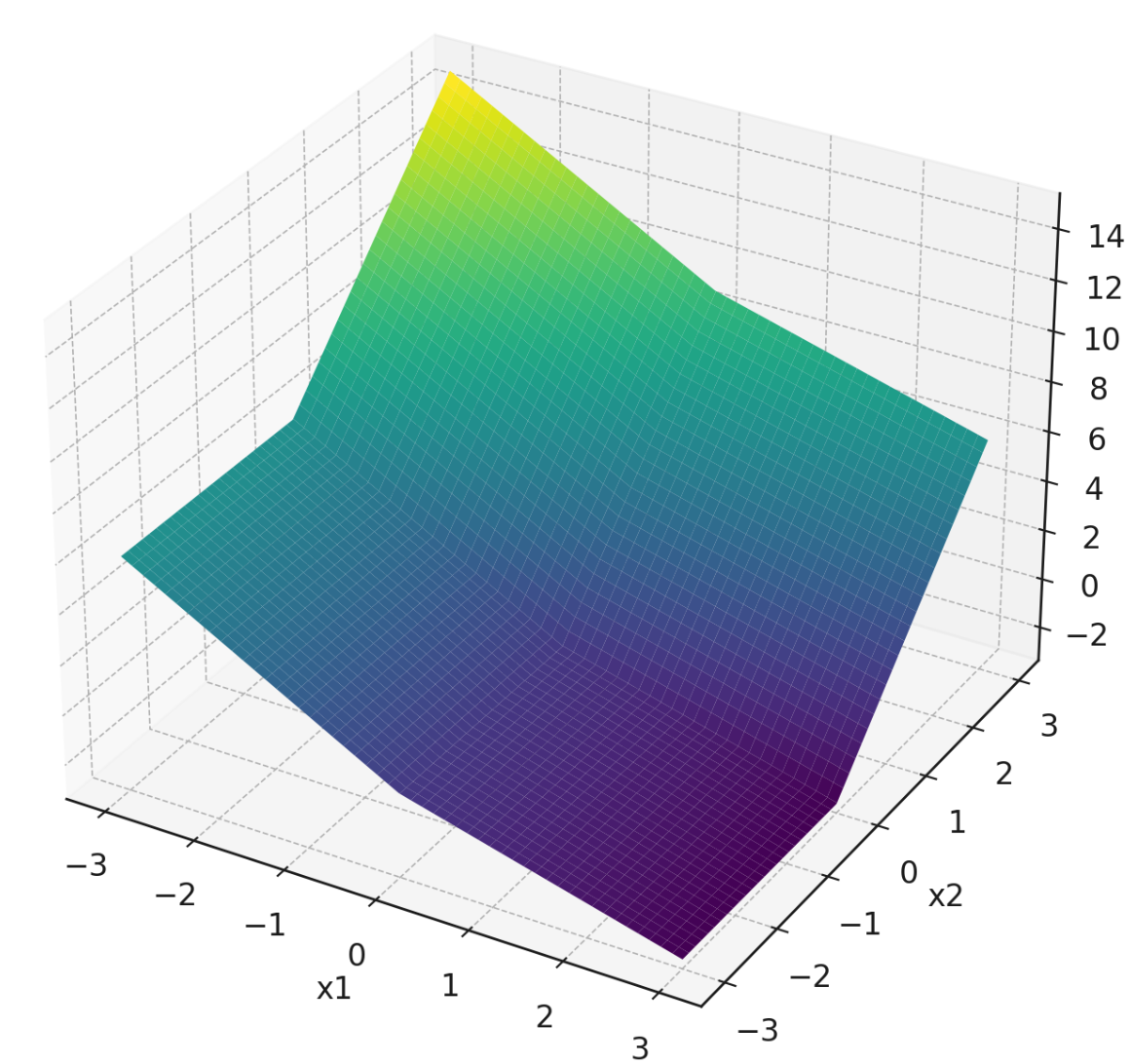
Note that the plot isn't intended to be precise enough to read off exact values, but it might help give you a sense of the function's behavior. In principle, you can do this question using only the piecewise definition of \(f\) given above.
Part 1)
What is the gradient of this function at the point \((1, 1)\)?
Part 2)
What is the gradient of this function at the point \((-1, -1)\)?
Part 3)
Which of the below are valid subgradients of the function at the point \((0,-2)\)? Select all that apply.
Solution
\((-2, 0)^T\) and \((-1.5, 0)^T\) are valid subgradients.
Part 4)
Which of the below are valid subgradients of the function at the point \((0,0)\)? Select all that apply.
Solution
\((-1, 3)^T\), \((-2, 0)^T\), and \((-1, 2)^T\) are valid subgradients.
Problem #085
Tags: gradient descent, subgradients
Recall that a subgradient of the absolute loss is:
Suppose you are running subgradient descent to minimize the risk with respect to the absolute loss in order to train a function \(H(x) = w_0 + w_1 x\) on the following data set:
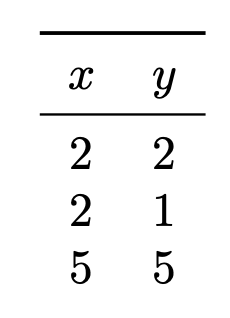
Suppose that the initial weight vector is \(\vec w = (0, 0)^T\) and that the learning rate is \(\eta = 1\). What will be the weight vector after one iteration of subgradient descent?
Problem #087
Tags: SVMs
Let \(\mathcal X\) be a binary classification data set \((\nvec{x}{1}, y_1), \ldots, (\nvec{x}{n}, y_n)\), where each \(\nvec{x}{i}\in\mathbb R^d\) and each \(y_i \in\{-1, 1\}\). Suppose that the data in \(\mathcal X\) is linearly separable.
Let \(\vec w_{\text{svm}}\) be the weight vector of the hard-margin support vector machine (SVM) trained on \(\mathcal X\). Let \(\vec w_{\text{lsq}}\) be the weight vector of the least squares classifier trained on \(\mathcal X\).
True or False: it must be the case that \(\vec w_{\text{svm}} = \vec w_{\text{lsq}}\).
Solution
False.
Problem #088
Tags: SVMs
Recall that the Soft-SVM optimization problem is given by
where \(\vec w\) is the weight vector, \(\vec\xi\) is a vector of slack variables, and \(C\) is a regularization parameter.
The two pictures below show as dashed lines the decision boundaries that result from training a Soft-SVM on the same data set using two different regularization parameters: \(C_1\) and \(C_2\).
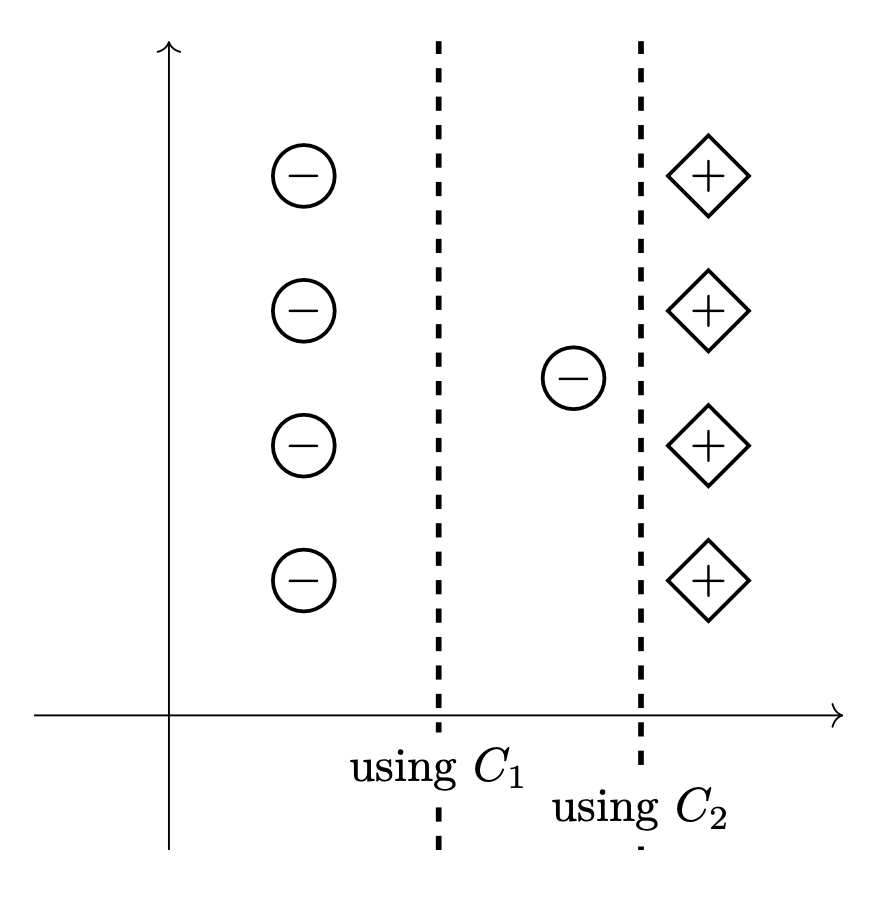
True or False: \(C_1 < C_2\).
Solution
True.
Problem #089
Consider the data set shown below. The points marked with ``+'' have label +1, while the ``\(\circ\)'' points have label -1. Shown are the places where a linear prediction function \(H\) is equal to zero (the thick solid line), 1, and -1 (the dotted lines). Each cell of the grid is 1 unit by 1 unit. The origin is not specified (it is not necssary to know the absolute coordinates of any points for this problem).
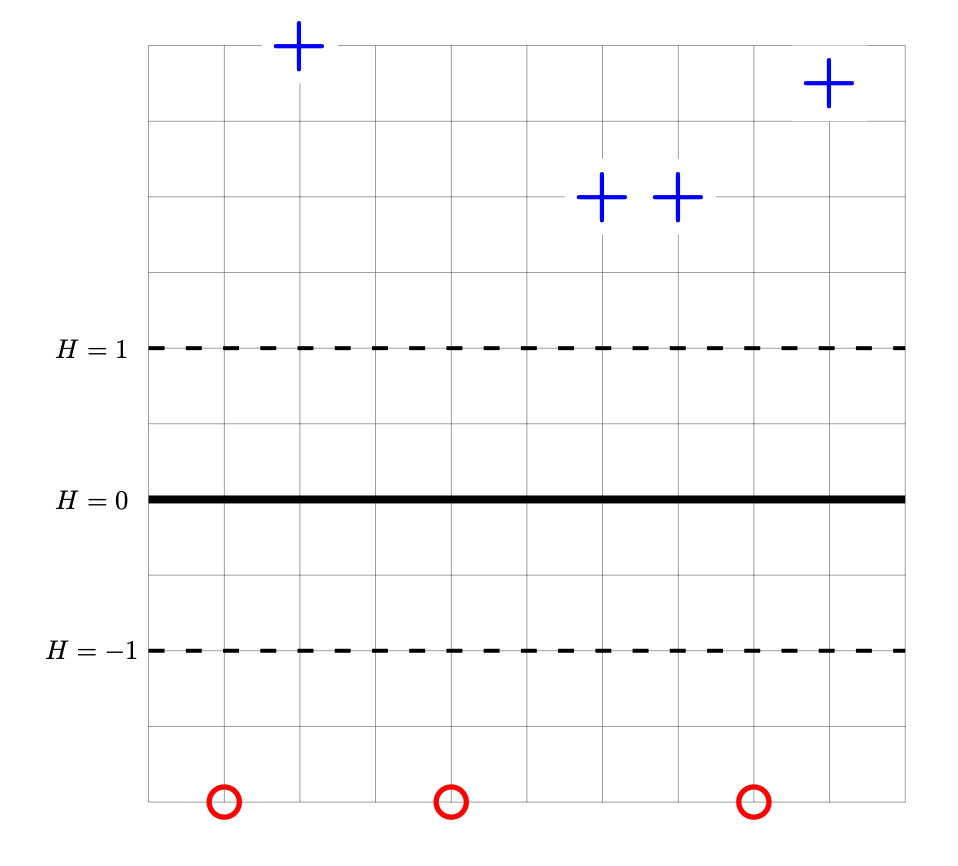
Let \(\vec w'\) be the parameter vector corresponding to prediction function \(H\) shown above. Let \(R(\vec w)\) be the risk with respect to the hinge loss on this data. True or False: the gradient of \(R\) evaluated at \(\vec w'\) is \(\vec 0\).
Solution
True.
Problem #090
Tags: gradients
Let \(\vec x, \vec\mu,\) and \(\vec b\) be vectors in \(\mathbb R^n\), and let \(A\) be a symmetric \(n \times n\) matrix. Consider the function:
What is \(\frac{d}{d \vec x} f(\vec x)\)?
Problem #103
Tags: covariance, object type
Part 1)
Let \(\mathcal{X}\) be a data set of \(n\) points in \(\mathbb{R}^d\), and let \(\vec\alpha\) be the solution to the kernel ridge regression dual problem. What type of object is \(\vec\alpha\)?
Solution
B.
Part 2)
Suppose \(\nvec{x}{1}, \ldots, \nvec{x}{n}\) is a set of \(n\) points in \(\mathbb{R}^d\), \(y_1, \ldots, y_n\) is a set of \(n\) labels (each either \(-1\) or \(1\)), \(\vec w\) is a \(d\)-dimensional vector, and \(\lambda\) is a scalar.
Let \(\vec\phi : \mathbb{R}^d \to\mathbb{R}^k\) be a feature map.
What type of object is the following?
Solution
D.
Part 3)
Let \(\nvec{x}{1}, \ldots, \nvec{x}{n}\) be a set of \(n\) points in \(\mathbb{R}^d\). Let \(\vec\mu = \sum_{i=1}^n \nvec{x}{i}\) be the mean of the data set, and let \(C\) be the sample covariance matrix.
What type of object is the following?
Solution
A.
Part 4)
Let \(\nvec{x}{1}, \ldots, \nvec{x}{n}\) be a data set of \(n\) points in \(\mathbb{R}^d\) sampled from a multivariate Gaussian with known covariance matrix but unknown mean, \(\vec\mu\). Let \(\mathcal L(\vec\mu)\) be the likelihood function for the Gaussian's mean, \(\vec\mu\). What type of object is \(\mathcal L\)?
Solution
Video explanation: https://youtu.be/wr8sNCEiIQs
Problem #118
Tags: object type
Part 1)
Let \(\mathcal{X}\) be a data set of \(n\) points in \(\mathbb{R}^d\), and let \(C\) be the sample covariance matrix of \(\mathcal{X}\). Let \(|C|\) denote the determinant of \(C\). What type of object is the following?
Solution
A.
Part 2)
Suppose \(\nvec{x}{1}, \ldots, \nvec{x}{n}\) is a set of \(n\) points in \(\mathbb{R}^d\), \(\lambda\) is a positive real number, \(\vec y \in\mathbb R^n\) is a vector of targets, and \(\vec\phi : \mathbb{R}^d \to\mathbb{R}^k\) is a feature map. Let \(K\) be the kernel matrix for this feature map on this data set, and let \(I\) be an identity matrix (the same shape as \(K\)). What type of object is the following?
Solution
C.
Part 3)
Let \(\nvec{x}{1}, \ldots, \nvec{x}{n}\) be a set of \(n\) points in \(\mathbb{R}^d\). Let \(\vec\mu = \frac1n \sum_{i=1}^n \nvec{x}{i}\) be the mean of the data set, and let \(C\) be the sample covariance matrix.
What type of object is the following?
Solution
B.
Part 4)
Let \(\nvec{x}{1}, \ldots, \nvec{x}{n}\) be a data set of \(n\) points, let \(\vec\alpha\in\mathbb{R}^n\), and let \(\kappa\) be a kernel function for a feature map \(\vec\phi : \mathbb R^d \to\mathbb R^k\). Suppose also that \(\vec z \in\mathbb{R}^d\). What type of object is the following?
Solution
A.